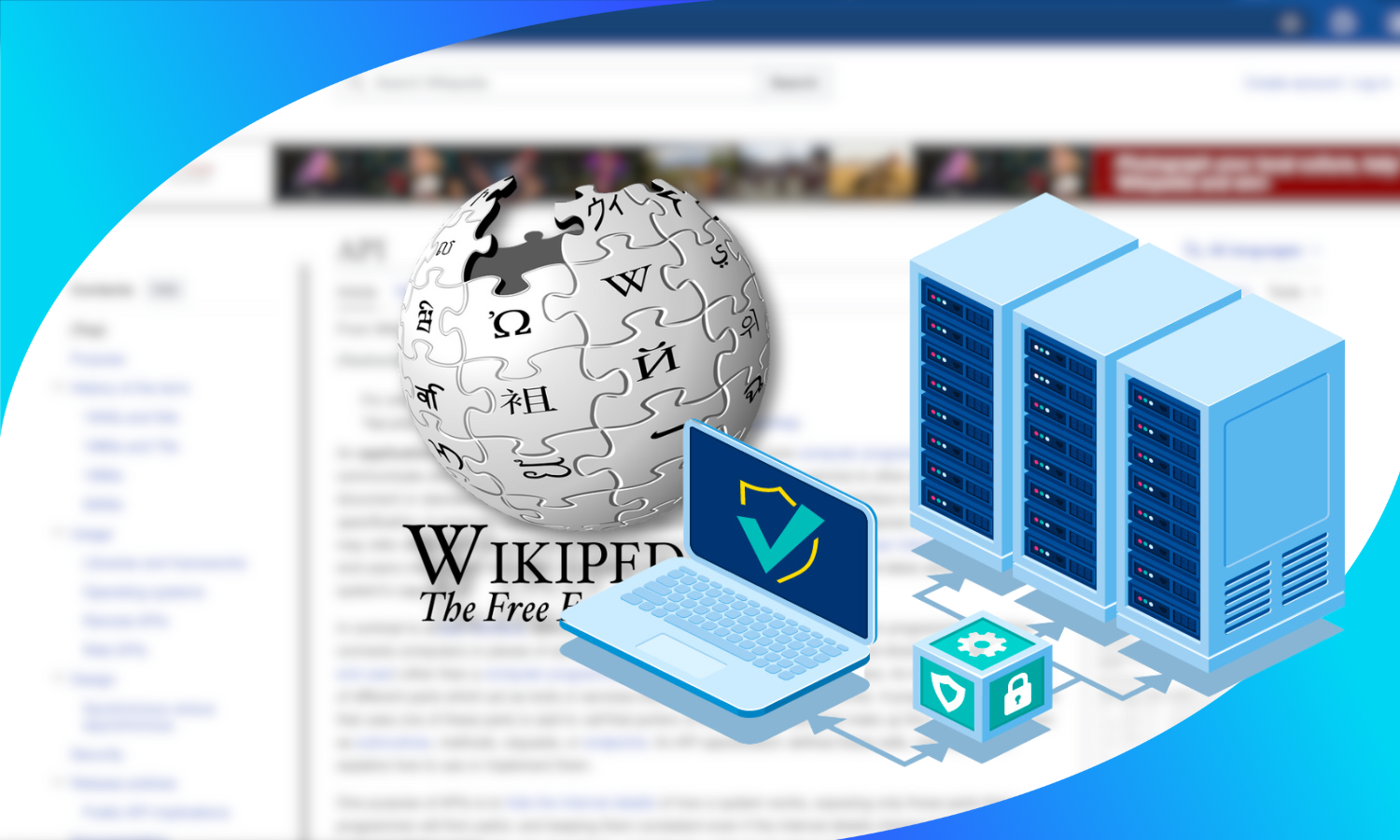
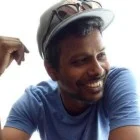
Updated · Feb 11, 2024
Updated · Oct 25, 2023
Meet Alex Williams, a seasoned full-stack developer and proficient technical writer. Graduating with... | See full bio
Florence is a dedicated wordsmith on a mission to make technology-related topics easy-to-understand.... | See full bio
Photo by Robin Glauser on Unsplash
Edge AI represents a significant revolution in modern computing, streamlining how organizations process and manage data. This innovative technology has emerged in unique use cases, including:
According to research, the value of the Edge AI market stood at $11.98 billion in 2021. It’s currently estimated to increase from $15.60 billion in 2022 to $107.47 billion by 2029. This accounts for a compound annual growth rate (CAGR) of 31.7% throughout the projected period.
In this comprehensive blog, you’ll uncover more about the revolutionary solution called Edge AI in detail, including how it works.
🔑 Key Takeaways:
|
Also called AI at the edge in some circles, Edge AI involves deploying artificial intelligence in an edge computing setting. It allows data processing to be closer to its collection source instead of relying on distant cloud computing facilities or external data centers to accomplish tasks.
To better understand how it works, dive into the key features of Edge AI in the following section.
Edge AI is a technological revolution in processing data and decision-making in various real-world scenarios and applications. In this network, devices and services collaborate seamlessly to generate and process data on the fly.
This kind of seamless interaction and the resulting immediate data-driven decisions are some of the hallmarks of Edge AI applications.
Some key features and reasons behind its effectiveness are as follows:
These powerful algorithms have been shown to help AI models learn and generalize how simple tasks are managed and carried out. Experts designed most Edge AI applications to accomplish specific tasks, with AI models able to recreate system-level behavior accurately.
💡 Did You Know? According to the latest statistics, global machine learning is expected to reach $21 billion in 2024. This number proves that ML is one of AI's most favored and successful sub-functions. |
Under Moore’s Law, parallel processing chips have evolved to enable energy-efficient operation and the deployment of intricate MLMs on devices that don’t consume much power. These dedicated devices at the network's edge can optimize computations without compromising performance.
Definition: Moore's Law is the observation made by the late Gordon Moore in 1965. The law claims that users can expect the speed and capability of computers to enhance every two years, though the value of those computers is halved. |
IoT devices have seen extensive adoption worldwide in recent years. Their high-speed connectivity and low energy requirements make them perfect candidates for executing AI algorithms at the edge of computing systems. This innovative approach eliminates the need to transfer data over the cloud constantly.
🎉 Fun Fact: Research revealed the number of connected IoT devices by 2030 will be 29 billion. This is approximately a 350% increase in a decade! |
Photo by Jorge Ramirez on Unsplash
Edge AI processes data at the collection source without transmitting it to cloud platforms. The hardware that processes this data is directly integrated with the input or sensor device. This method speeds up the data processing and analysis, increasing the overall efficiency of the application.
The illustration shows that devices send metadata to the cloud for advanced post-processing and analytics. If the data captured is fundamental, it will also be sent to the cloud, with decisions still made in real time.
Regardless, AI at the Edge doesn’t require devices to continuously send data to the cloud, improving the cost and speed of transferring it.
📝 Note: When implementing AI at the Edge, you must clearly understand the task you’re deploying it for and the output you’re expecting, as this will help you build the most robust model possible. |
Artificial intelligence has rapidly expanded in scope and capability over recent years, generating massive troves of data. If analyzed properly, this data can provide valuable insights into the industry to which it belongs. These AI systems require the following to help the models function effectively and deliver higher-quality results:
As Artificial Intelligence grows, systems must be able to handle all computations and data without latency or lag. This is where Edge AI shines. It provides advanced analysis and data collection closer to the generation source instead of relying solely on a cloud-based solution.
Processing data on the device or nearby it instead of transmitting it reduces latency and improves the speed of applications. It also offers various benefits, which you’ll learn in the section below.
As a true revolution in computing, Edge AI offers several benefits to organizations of all sizes across various industries and sectors. Some critical benefits of Edge AI include:
A primary modern use of Edge AI systems is in the manufacturing sector. As a result, manufacturers of all sizes have begun to modify their processes by employing AI at the edge.
Here are some use cases related to Edge AI:
This is where device sensor data is utilized to discover potential problems as soon as possible and anticipate when a machine may need repairs. Edge AI helps treat any issues immediately while minimizing production downtime.
Many marketers use Edge AI as a tool to facilitate online shopping with the following services:
Likewise, Edge AI-driven chatbots can provide more relevant customer service by adapting in real-time to the customer's queries and tone, all while analyzing data locally on the user’s device.
Another great use of Edge AI in the real world is how it can help revolutionize workflows by assisting professionals to automate more tedious aspects of their jobs using Edge AI-assisted apps.
For instance, automating document comparisons between PDF, Word, and Office formats can be a lifesaver for busy professionals. Utilizing Edge AI-assisted apps for document comparison helps with a lot of things, such as:
These key features help demonstrate what the future workday will look like and what the greater world will look like with Edge AI.
While the various applications of AI at the edge can benefit businesses and organizations of all sizes, it’s not without its potential challenges.
Organizations may need help deploying and managing the AI models used in their edge devices. As a result, it can be challenging to ensure that models perform correctly across all devices.
As an emerging concept in computing, there simply aren’t enough people with the experience and knowledge to facilitate everything that goes into Edge AI. An expert must know how to build and optimize models for Edge devices alongside a general uncertainty surrounding the broader implications of using these devices.
Edge AI devices are often situated in public or semi-public areas, making them vulnerable to physical cyber attacks. These devices also often manage sensitive data, increasing the threat of data breaches.
✅ Pro Tip: To mitigate these risks, you must have:
|
With many devices and platforms available, ensuring that various components work together seamlessly can be challenging. Adopting open standards and protocols is essential, as it reduces the risk of vendor lock-in and promotes interoperability.
To ensure easy integration, you can work closely with stakeholders, including:
Despite the challenges shown in the previous section, the future of Edge AI still holds plenty of promises going forward. Here are two major things to expect in the future:
AI at the Edge has only begun to emerge in real-world applications and scenarios. The future has the potential for many more innovative and transformative advancements.
Many industries and companies already utilize Edge computing and AI in conjunction. It won’t be long before more businesses adopt them fully.
As generative AI becomes more standardized, people will see a particular focus on processing at the edge. After all, many don’t own massive cloud spaces for processing data. They will require generative AI to work efficiently and effectively where they are.
That said, in a world where technology is fast-moving, older methods will be replaced in favor of Edge Computing and Edge AI. As a result, others will adapt to incorporate these new technologies and their benefits.
One Edge AI example is real-time traffic updates on autonomous vehicles, smartphones, and connected devices.
Edge learning is a subset of AI in which data processing occurs “at the edge” of the data's origin using a trained set of algorithms.
If you want to be an AI edge engineer, Microsoft offers training where you’ll learn about creating solutions using IoT and the cloud, exploring the use of DevOps for edge devices, and many more.
Your email address will not be published.
Updated · Feb 11, 2024
Updated · Feb 11, 2024
Updated · Feb 08, 2024
Updated · Feb 05, 2024